Munjal Shah’s company is taking a cautious approach to launching its flagship nondiagnostic healthcare LLM.
Hippocratic AI launched in 2023 with a laser focus on nondiagnostic healthcare large language models. The goal was to provide artificial intelligence assistants that can help with everything from patient education to appointment scheduling, but won’t try to diagnose or recommend treatment plans. Just over 18 months later, the company is nearing that goal, announcing that its first product — a GenAI-powered staffing marketplace — entered a third and final round of safety testing in March.
“You can’t have an AI autopilot doing diagnoses. It’s too dangerous,” says Munjal Shah, the company’s founder and CEO. “But there’s so much more to healthcare than diagnoses.
“What if, instead of building an AI co-pilot for diagnosis, we do autopilot for low-risk tasks? What if we build fully automated AIs that call people on the phone and talk to them and do preoperative calls before your colonoscopy to make sure you’re taking the drink you need and check in with you to make sure you’re getting that MRI you were supposed to get done, that you keep blowing off?”
Safety and Training
A cautious, specialized approach sets Hippocratic AI apart in a field where companies are racing to apply generalist AI models to all kinds of applications, sometimes with troubling results. Reports of ChatGPT and other LLMs confidently presenting inaccurate information as true and giving biased or problematic advice highlight the risks of deploying these powerful but imperfect systems in healthcare without proper guardrails.
Hippocratic AI’s solution, along with focusing on low-risk applications, is a multipronged strategy combining carefully curated training data, specialized model architectures, and rigorous testing and validation by medical professionals to build healthcare-specific LLMs.
The company’s flagship product is what it calls a “constellation” of AI models, each specializing in different healthcare tasks. At the center is a primary conversational agent trained to handle general patient interactions. This central model is supported by a team of specialist models — one for medication information, another for lab results, others for nutrition advice or hospital policies, and so on.
This distributed approach allows for more focused training and easier updates to individual components. It also provides multiple layers of fact-checking, as the specialist models can verify or correct information from the primary agent.
But perhaps most notable is Hippocratic AI’s emphasis on safety and real-world testing. The company puts its models through a gamut of evaluations involving thousands of nurses, doctors, and other healthcare professionals. These experts assess the AI’s performance across a range of metrics, from medical accuracy to bedside manner.
“We actually have thousands of nurses interacting with our large language model as if they’re patients and it’s the chronic care nurse,” says Munjal Shah. “And they basically do a blind taste test where they don’t know if they’re talking to a nurse or an AI. Only when they think it’s safe will we launch.”
The Broader Context
Hippocratic AI’s focus on practical, nondiagnostic applications and rigorous safety testing places the company at an interesting inflection point in the broader landscape of AI in healthcare.
A recent Nature article outlined both the tremendous potential and significant challenges facing LLMs in medicine. The authors pointed to empirical work showing that these models could democratize access to medical care, assist with research and education, and free up clinicians’ time by handling routine administrative tasks. But they also noted the risk of spreading misinformation, exacerbating biases, and potentially eroding critical thinking skills if relied upon too heavily.
Shah points out that, with a focus on nondiagnostic applications, Hippocratic AI is well positioned to avoid these risks. And its emphasis on transparency and expert validation aligns with growing calls for greater scrutiny of AI systems in healthcare and other sectors.
“You want to make sure it has all the healthcare knowledge it needs, so we’ve actually taken the time to do that,” he explains. “You want to make sure you work with health systems to ensure it’s safe, so you build it with them, not outside of them, which we also did.”
Hippocratic AI has carried out a three-phase approach to certifying the safety of its LLMs. Phase 1 involved leading experts and physicians in AI safety reviewing the initial product and providing reinforcement learning through human feedback. For Phase 2, over 1,000 U.S.-licensed nurses and doctors tested the LLM. After these healthcare workers deemed the product safe, the company advanced to Phase 3, in which over 40 health system and payor partners and 5,000 healthcare workers are testing the product.
“Since the founding of Hippocratic AI a year ago, the company trained its foundational model, achieved a key safety threshold, identified novel use cases to solve the healthcare staffing crisis, and established partnerships with 40 health systems, payers, and digital health companies,” said Hemant Taneja, CEO and managing director at General Catalyst, one of the investors behind Hippocratic AI’s $120 million in funding.
“Hippocratic AI has grown rapidly due to sound execution and strategy while prioritizing safety. The company is a leading standard of radical collaboration at work and will play a critical role in transforming our healthcare system to make it more proactive, affordable, and equitable.”
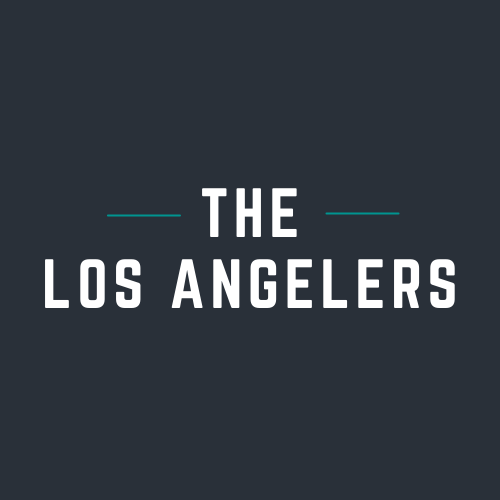
Comments are closed.